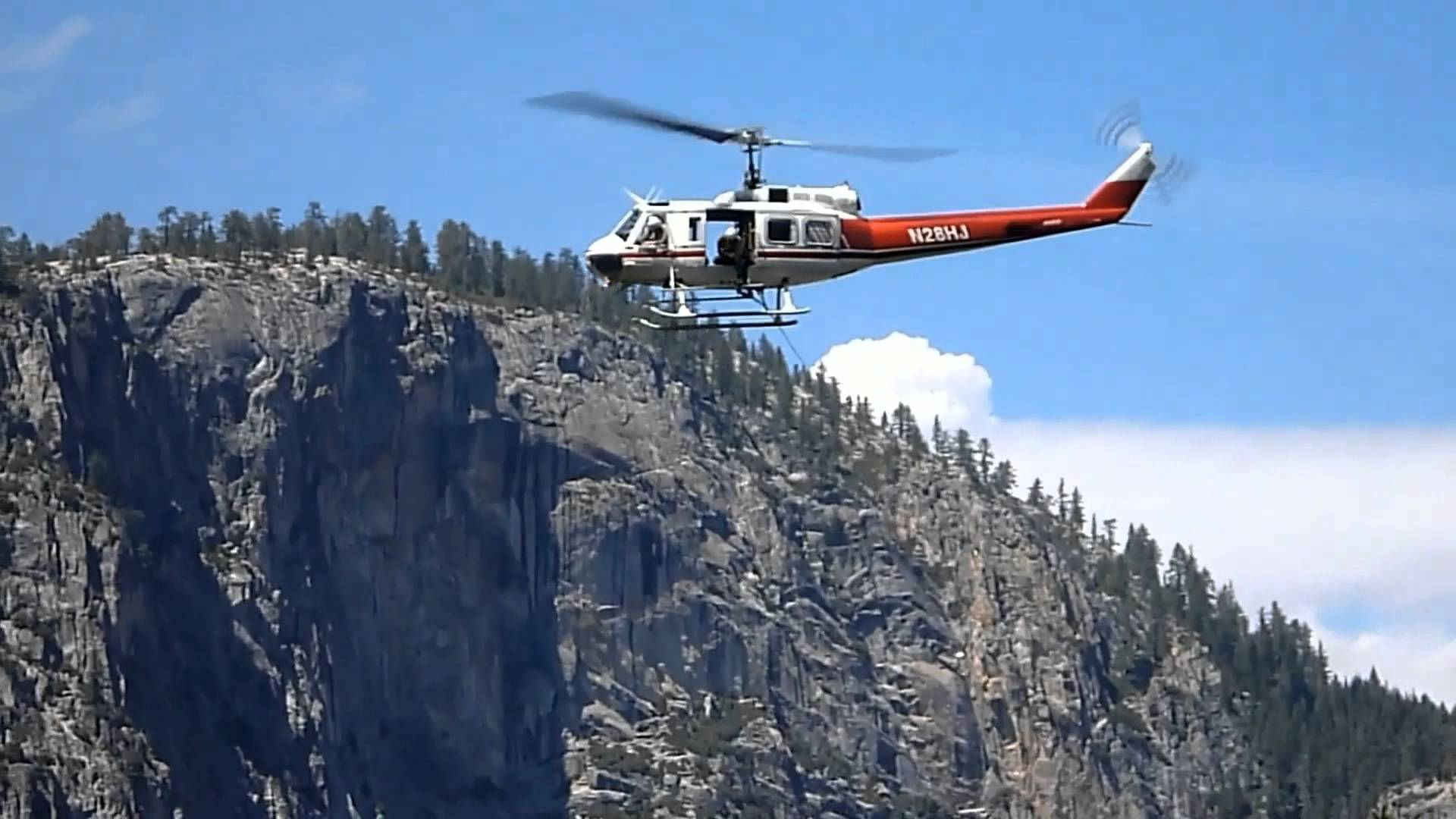
Yosemite Analytics
Yosemite Analytics Viewpoint
BI Solution Strategy
The most value is provided by a BI application developed using 3 approaches: a company's specific analytic requirements, industry standard metrics, and data warehousing.

Company Analytic Requirements
BI applications are often built to satisfy a small but well-defined set of requirements, usually represented by company-provided charts or scorecards. This provides a good contractual vehicle where the BI developer can demonstrate that they have satisfied the company's needs. However, some shortcomings of this approach are:
· The company's needs evolve as soon they see the first dashboard, leading to many iterations.
· Companies always start at square one and often never realize full analytic capabilities.
· Some companies focus on special cases of generic metrics that would provide more value.
· There may not be just one single company, such as when an analytic application is developed
to support another product, like QuickBooks™.
Industry Standard Metrics
A company's analytic requirements can be augmented by industry standard metrics. There are many published and online sources containing standards for financial, sales, and supply chain metrics. As an observation, everyone understands year-to-date revenue but some people confuse margin and return on investment (ROI).
In addition, not all obvious metrics are actionable. Other than "make more money," what action is prescribed if year-to-date revenue is lower than expected? The supply chain world has the concept of a perfect sales order, which does not normally surface as a company analytic requirement. A perfect sales order is one which: is satisfied in one pick (a manufacturing term), has no revisions, is completed on time, has no credit returns, and so forth. Perfect order failures indicate which business process is not performing.
Data Warehousing
Very large data warehousing efforts, dating back to the 90’s, failed to provide end-user value. These data-centric approaches provided rigorous definitions of what constituted a code versus a number versus an ID but failed to provide needed analytics - and therefore needed answers. Better data-centric modeling is possible if it doesn’t focus on relational database structures but identifies the star schemas that capture real world events, such as sales orders or manufacturing orders or shipping orders. Still, looking at data alone does not capture important relationships between events, such as when did each sales order ship or what is the difference between list price and sales price.
The data-centric approach is useful when layered with other approaches. Birst, in particular, has the capability to store many parameters that would not normally appear on a dashboard but are useful for drill-down analyses, such as: sales order creation date vs. issue date vs. manufacturing date vs. first ship date (etc.), hour sold (for retail), sales order status, sales order item status, and many more. Unlike a hiker who needs to think very carefully about the size and weight of each item they put into their backpack, using the data-centric approach to BI we can add these additional parameters to the data model at negligible cost to the overall project, and we will be much better prepared to handle unexpected conditions and user requests as they occur.
Our blended approach helps ensure that people will be able to get the answers they need when they need them.
Contact us today to discuss your BI goals and how we can help get you there safely and successfully.

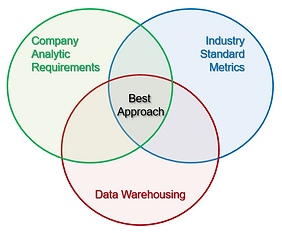